Generative AI: Unlocking Creativity with Artificial Intelligence
The Rise of Generative AI: Unlocking Creativity with Artificial Intelligence
Artificial Intelligence (AI) has transformed numerous facets of our lives, from automating tasks to enhancing decision-making processes. Among the most fascinating advancements in AI is Generative AI, a subfield focused on creating new and original content. Whether it’s generating realistic images, writing compelling stories, or composing music, Generative AI is redefining the boundaries of creativity and technology. In this article, we will explore what Generative AI is, how it works, its applications, challenges, and its potential impact on the future.
What is Generative AI?
Generative AI refers to a class of algorithms that can produce content, such as text, images, audio, or even code, by learning patterns from existing data. Unlike traditional AI models that analyze or classify data, Generative AI creates new data that resembles the input it was trained on. This capability has opened up exciting possibilities in fields ranging from art and entertainment to medicine and design.
At its core, Generative AI is about using machines to mimic human creativity. The goal is not just replication but innovation—producing outputs that are novel yet contextually appropriate.
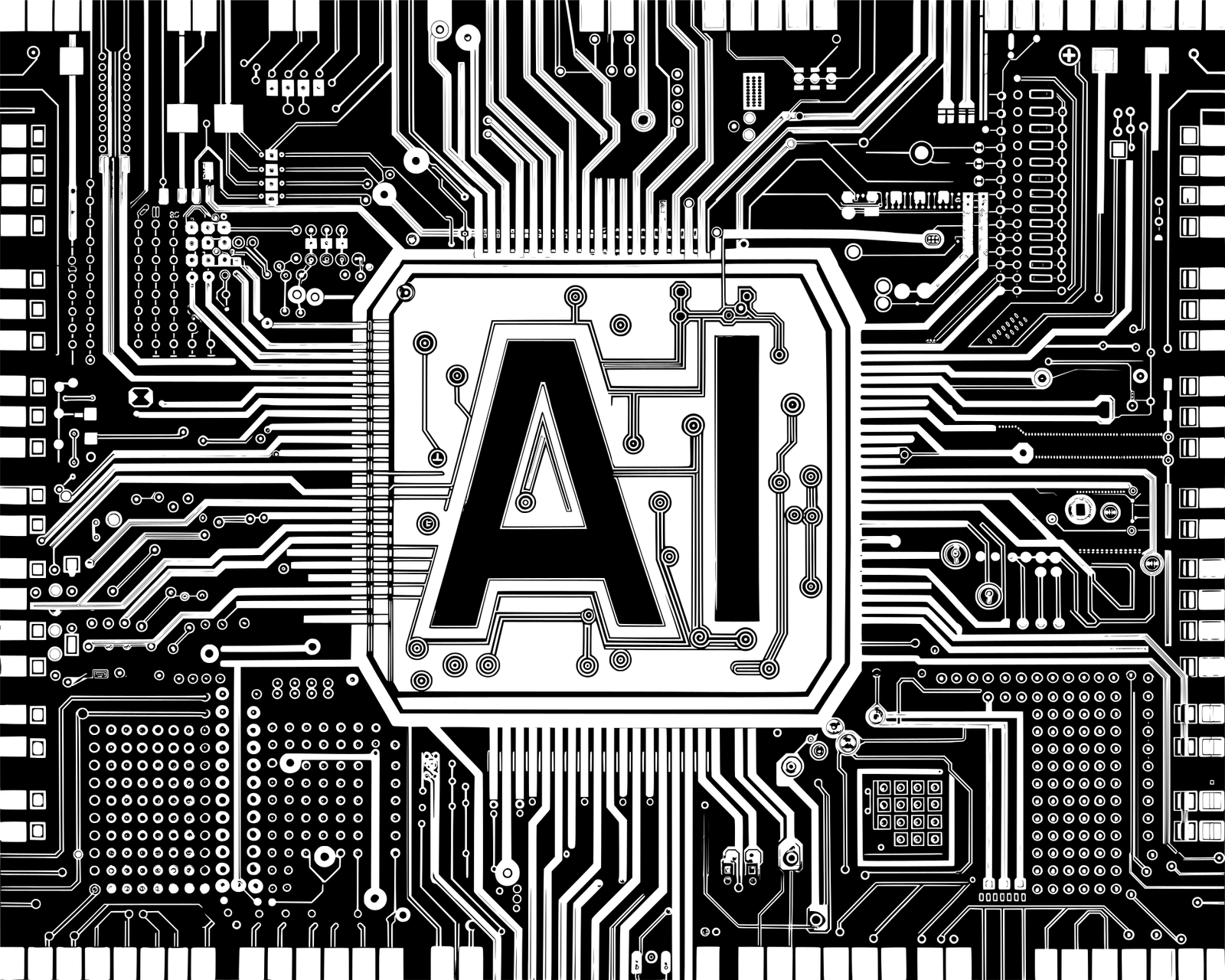
How Does Generative AI Work?
Generative AI relies on advanced machine learning techniques, particularly deep learning. Here are some of the key components and methods involved:
- Neural Networks: Generative AI models are often built on neural networks, which are inspired by the structure and functioning of the human brain. These networks process data through interconnected layers, enabling the model to learn complex patterns.
- Generative Adversarial Networks (GANs):
- GANs consist of two neural networks: a generator and a discriminator.
- The generator creates new data (e.g., an image), while the discriminator evaluates its authenticity by comparing it to real data.
- Through an iterative process, the generator improves its outputs until they are indistinguishable from real data.
- Transformer Models:
- Transformer architectures, such as GPT (Generative Pre-trained Transformer), are widely used for text generation tasks.
- These models rely on self-attention mechanisms to understand and generate coherent sequences of text.
- Variational Autoencoders (VAEs):
- VAEs are another method used in Generative AI. They compress data into a lower-dimensional representation and then reconstruct it, generating new variations in the process.
- Diffusion Models:
- These models are increasingly used for generating high-quality images. They work by reversing a noise-adding process to produce realistic visuals.
Training these models requires large datasets, high computational power, and extensive fine-tuning to ensure accuracy and relevance.
Applications of Generative AI
Generative AI has diverse applications across industries, showcasing its versatility and potential. Here are some prominent use cases:
- Content Creation:
- Writers and marketers use Generative AI tools to create articles, social media posts, and advertisements. These tools can help with idea generation, saving time and effort.
- Art and Design:
- Generative AI enables artists to create unique visuals and designs. Platforms like DALL-E and MidJourney allow users to generate images based on textual descriptions, opening new avenues for digital art.
- Healthcare:
- In medicine, Generative AI is used to design new drugs, predict protein structures, and create synthetic medical images for training purposes.
- Gaming and Entertainment:
- Generative AI creates game assets, characters, and even storylines, enriching the gaming experience. It is also used in music composition and film production.
- Fashion and Retail:
- In the fashion industry, Generative AI is employed to design clothing, accessories, and even virtual try-on experiences for customers.
- Education:
- Generative AI helps create personalized learning materials, quizzes, and simulations, enhancing the educational experience.
- Coding and Software Development:
- Developers leverage Generative AI to write, debug, and optimize code. Tools like GitHub Copilot assist programmers by suggesting lines of code in real time.
Advantages of Generative AI
- Creativity Boost:
- Generative AI expands the horizons of creativity, enabling individuals and organizations to explore new ideas and concepts.
- Time Efficiency:
- By automating content creation, Generative AI reduces the time required for repetitive or labor-intensive tasks.
- Cost-Effective Solutions:
- It eliminates the need for manual effort in areas like graphic design, writing, and prototyping, leading to cost savings.
- Personalization:
- Generative AI creates tailored solutions, from personalized marketing campaigns to customized educational materials.
Challenges and Limitations
While Generative AI holds immense promise, it is not without challenges:
- Data Dependence:
- The quality of outputs depends on the quality and diversity of training data. Biased or incomplete datasets can lead to flawed results.
- Resource Intensity:
- Training Generative AI models requires significant computational resources, making it expensive and environmentally taxing.
- Ethical Concerns:
- The misuse of Generative AI for creating fake news, deepfakes, or malicious content poses serious ethical dilemmas.
- Lack of Originality:
- Although Generative AI can produce novel outputs, it relies heavily on existing data, raising questions about true originality and creativity.
- Regulation and Accountability:
- As Generative AI becomes more powerful, there is a need for clear regulations to address its ethical and societal implications.
Ethical Considerations
The rise of Generative AI has sparked debates around its ethical use. Key concerns include:
- Misinformation:
- Generative AI can be exploited to create misleading or harmful content, such as doctored images or fabricated news stories.
- Intellectual Property:
- The use of existing data to generate new content raises questions about copyright and intellectual property rights.
- Bias and Fairness:
- Developers must address biases in training data to ensure fair and equitable outcomes.
- Job Displacement:
- The automation of creative tasks could impact jobs in industries like writing, design, and entertainment.
The Future of Generative AI
As technology advances, the potential of Generative AI will continue to grow. Researchers are working on creating more efficient models that require fewer resources and are less prone to biases. Collaboration between industry and academia will likely drive innovation, leading to new applications and use cases.
The integration of Generative AI into everyday life also necessitates the development of frameworks for ethical and responsible use. Balancing innovation with societal impact will be crucial to ensure that Generative AI benefits humanity as a whole.
Conclusion
Generative AI represents a groundbreaking frontier in artificial intelligence, blending creativity with technology. Its ability to generate realistic and original content has far-reaching implications across industries and disciplines. While challenges remain, the opportunities it presents are vast and transformative. By understanding and leveraging Generative AI responsibly, we can unlock its full potential and shape a future where humans and machines collaborate to drive innovation.
What are your thoughts on Generative AI and its impact on creativity? Do let us know in comments. 😊